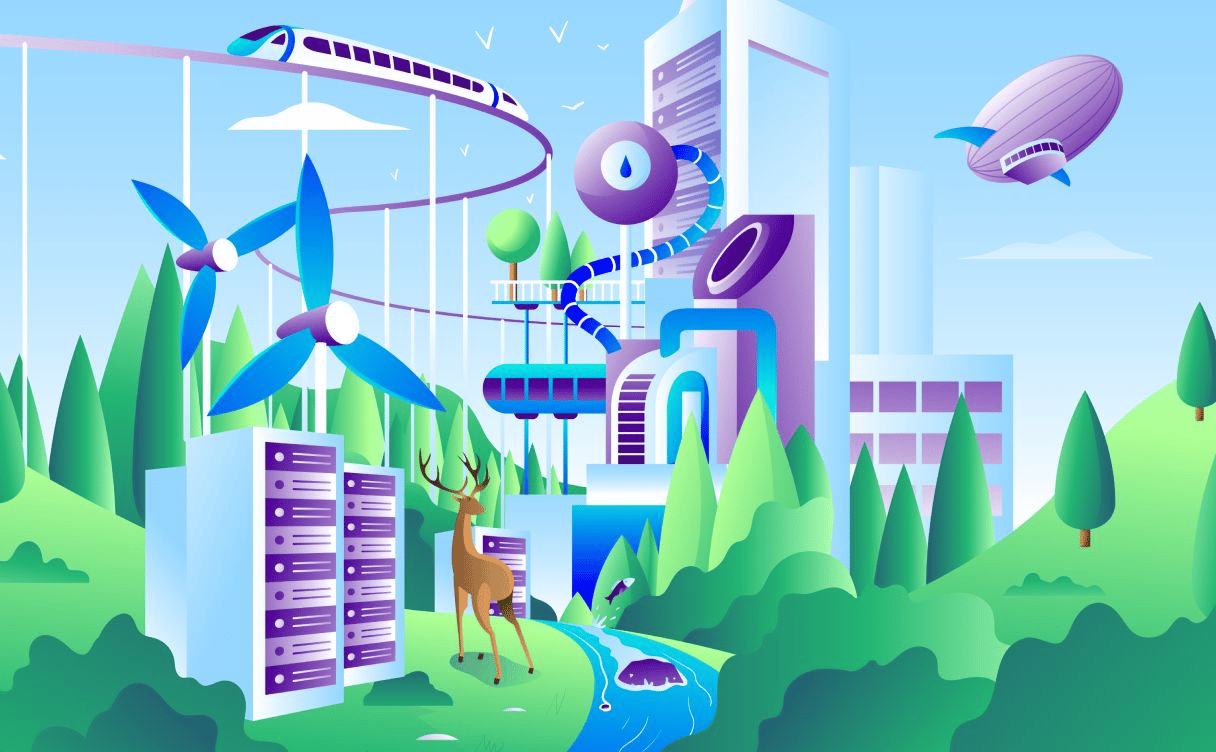
How sustainable is your cloud provider?
Today, cloud service providers (CSPs) are tied in a ‘sustainability race’, all vying to claim their solutions have the least impact on the environment.
Tech is permeating every nook and cranny of healthcare and medical research. From remote consultations to AI-assisted diagnoses, recent years have seen Medtech innovations gain widespread adoption at unprecedented haste.
There are 3 principal reasons for this.
For one, the pandemic accelerated the development, demand, and adoption of telemedicine and other digital healthcare solutions. Strides in this area were made possible by advances in connectivity.
Simultaneously, next-gen tech is finally finding traction in medicine, as Artificial Intelligence (AI) solutions mature into practical, commercially-viable tools.
Cloud is the third piece of the puzzle. By enabling seamless and affordable tech integration and safe operations for hospitals, while also arming ambitious startups with the immense computing power required to develop tomorrow's solutions, the cloud is making it viable to put the tech into practice.
All of this is great news for everyone involved. Patients get more accurate diagnoses, easier access to specialist insights, and faster time to treatment. Doctors benefit from improved workflows and having to spend less time on menial tasks. And medical institutions see higher efficiency while minimizing the risk of litigation arising from misdiagnoses.
All in all, we're in the initial stages of a new era of healthcare.
Let's take a look at how exactly it is taking shape.
Automated robot surgeons have made waves in popular media. Surgeons already use robotic arms for high-sensitivity procedures, but, when imbued with AI, those robotic arms become even more precise tools that enhance the work of doctors by, for example, improving instrument positioning during surgery, which leads to better and more consistent outcomes.
Still, the reality is that robot surgery is a niche use of AI in medicine. AI is mostly used to analyze data – be it in the form of databases or images. While this may seem less glamorous, it has a broader impact.
One of the most widespread uses of AI in healthcare is in medical image analysis. AI can be trained to, for example, analyze a chest X-ray and identify any abnormalities in order to spot cancerous growths. In fact, it has been trained to do just that and existing solutions are already outperforming specialized radiologists in terms of accuracy. That said, it's important to note that the best results are attained when a specialist works in tandem with AI.
From chest X-rays and mammography to brain scans and bone fracture detection, AI enhances the specialist's ability to detect issues that might otherwise slip by unnoticed. Earlier detection leads to faster treatment and a better chance of recovery.
Here's how the AI is trained for X-ray analysis: it's fed hundreds of thousands, even millions, of high-quality X-rays that are complemented with data that tells it what's going on in the picture. Thanks to such large datasets it learns to recognize patterns and identify issues.
And therein lies a major challenge for developing AI solutions – beyond the technical development, it can be extremely difficult and time-consuming to gather sufficient 'learning material' for the algorithm.
Each image you feed the AI must be high-quality and hand-annotated with all the necessary information. More than that, the data labeling should involve review by multiple specialists, as fringe cases often spark professional debate.
Privacy laws also raise further problems in terms of data access, as the legality of sharing sizable patient record databases with external parties has been and will continue to be cause for public concern and legal action.
These issues are well understood and, among other things, such as the elimination of bias in data, are actively being worked on. Scientists hope to deal with these challenges by developing novel techniques and building better models.
These reasons reveal why it takes years to bring a solution to market. Still, usually, it's a solution worth waiting for, as the positives extend far beyond simply more accurate diagnoses for patients.
A major plus is improved accessibility to medical insights, as non-specialists can use AI to make crucial early decisions. Particularly valuable in regional hospitals where it doesn't make sense to have a full-time specialist, AI allows doctors to triage patients and only escalate cases where specialist insight is truly needed. This triage also allows specialists to minimize menial work and focus on the most pressing cases first.
The commercial viability of solutions such as the ones described above is closely tied to the cloud.
If every institution had to host such resource-intensive software locally, then between server deployment and maintenance requirements, the expenses would be astronomical and it's unlikely that the broader population would ever benefit from such tech developments.
Instead, the cloud makes it possible for the solutions to be hosted in a single location that the participating institutions access via a proxy server that's quick and easy to set up on the hospital's existing infrastructure.
This proxy server also plays a key security role. To be GDPR-compliant and ensure sensitive patient data isn't sent to a foreign nation, the server anonymizes it by scrubbing it of all personal information, except for that which is required for the diagnosis, e.g. patient age. Once the image is returned after the AI has analyzed it, the details are added back onto the file to ensure specialists can work with it.
While the cloud makes modern healthcare solutions usable, it also does a more fundamental thing – it makes them possible. Similar concerns about expenses apply to the startups that look to create these next-gen AI solutions. Requiring significant computing power, sizable investments in hardware are needed to create the necessary environment for developing such solutions.
Accordingly, startups will typically choose to turn to cloud service providers to gain the necessary computing resources and avoid risky upfront investments, particularly in their early stages of development.
As it stands, cloud service providers are playing a central role in bringing to life current and future digital healthcare solutions, in terms of both research and deployment.
While cost efficiency is by far the biggest benefit CSPs bring to the table, they also play an invaluable role in ensuring security in an industry that works with extremely sensitive data. CSPs will have to continue to manage soaring demand, as healthcare becomes a permanent fixture on the growing list of industries that depend on the cloud for their daily operations, as well as evolution.
Still, cloud providers will have to step up to this challenge by offering increasingly energy-efficient solutions. As the computing power required for training advanced AI models grows, so does the strain this puts on the environment.
Fortunately, the environmental impact can be significantly lessened through the use of energy-efficient data centers that are assembled in such a way as to minimize energy and water use through state-of-the-art space design and highly-efficient cooling systems, among other things.
For instance, Scaleway's energy-efficient DC5 data center can reduce the energy used by complex AI computations by at least 23% – saving hundreds, possibly thousands, of MWh of energy. Additionally, external research suggests that how and where we train neural networks can reduce the associated carbon footprint by up to 1000x.
One can only hope that the people looking to ensure better health for all are cognizant of the broader impact of their actions and don't sacrifice long-term perspective for short-term gains. After all, as they say, “the cure can't be worse than the disease”.
Today, cloud service providers (CSPs) are tied in a ‘sustainability race’, all vying to claim their solutions have the least impact on the environment.
In this article, we will talk about active learning and how to apply the concepts to an image classification task with PyTorch.
Message Queuing Telemetry Transport (MQTT) is a publish/subscribe messaging protocol that allows two remote devices to communicate via messages asynchronously with low bandwidth.