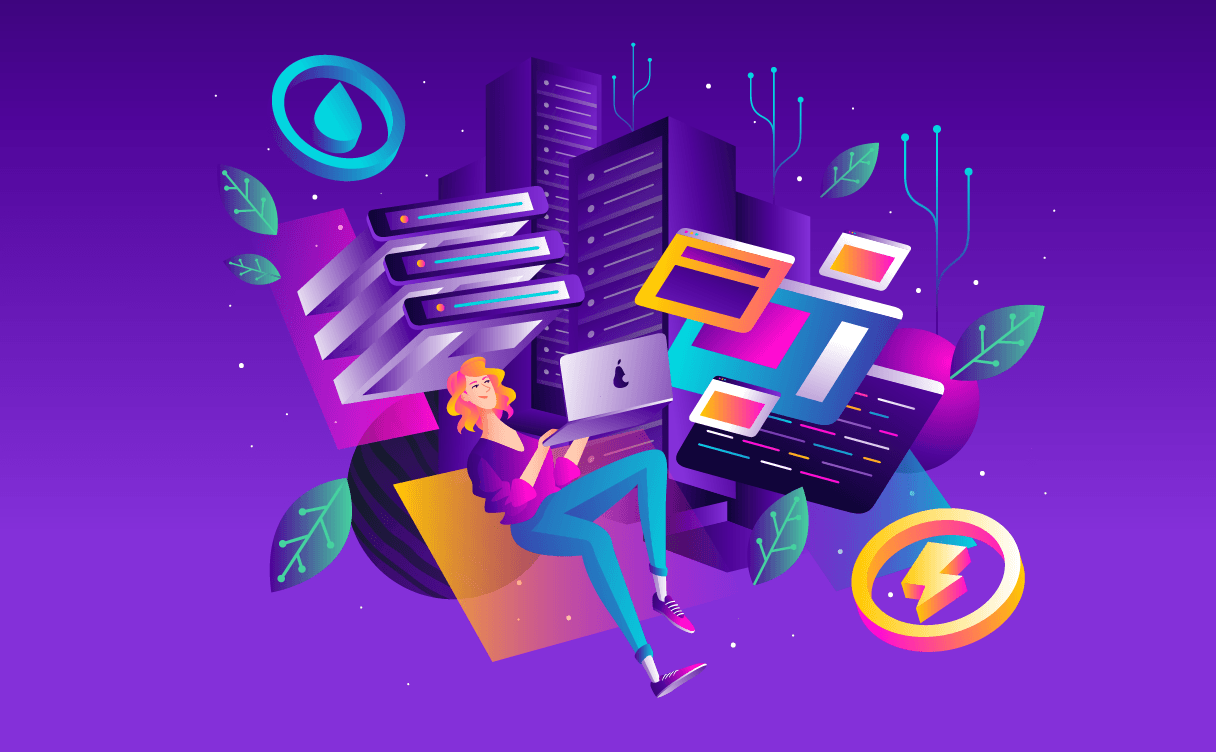
How can engineers make IT more sustainable? Part 4: What to do next
What is next for Green IT? Regulation, and 2050 carbon neutrality targets, will drive a shift from nice-to-have to must-have. And then there's AI...
Just over a year after the ChatGPT-fuelled generative AI explosion, it’s hard to remember a time without these groundbreaking tools. However, it remains to be seen if the breakneck speed of change has given us enough time to fully assess generative AI’s true impact on the planet. So let’s take a look.
First and foremost, it’s now well established that generative AI requires considerably more computing power than standard calculations. A key reason for this is that generative AI model training calls for GPUs rather than CPUs. The former generally requires around four times more energy than the latter (case in point: Ampere’s CPUs for AI consume 3-5 times less energy than the equivalent NVIDIA machines).
Furthermore, as AI GPUs tend to generate 2.5x more heat than CPUs (standard CPUs used in cloud computing are in the range of 250-350W TDP, whereas GPUs are in the 750-800W range, cf. Intel, AMD x2, & NVIDIA), they require that much extra cooling power. So the processors needed for generative AI training and inference are considerably more power-hungry than pre-generative AI models.
Then there’s the difference between training and inference. Looking at the former, or the process required to ‘educate’ a generative AI model by feeding it as much data as possible, the emissions generated by training vary hugely depending on the model:
(tCO2e = tons of CO2 equivalent, namely CO2 + the 3 other most potent greenhouse gasses)
This means that training a generative AI model can generate anything from the equivalent of three French people’s annual emissions (10 tCO2e), to 50.
But of course, training is a one-off occurrence. Inference, or the everyday usage of a model, has its own impact, which has been estimated at 200 times higher than that of training. According to French tech association Data for Good, considering ChatGPT has 100m weekly users, that’s 100,000 tCO2e/year for GPT-3.5.
To put it another way, generating one image with generative AI can use as much energy as that required to fully recharge a smartphone, according to the latest white paper co-authored by Sasha Luccioni, Climate Lead and AI Researcher at Hugging Face. "Can" is the operative word here, however, as The Verge points out, given the huge variety of GenAI models already available.
Then there’s water. Also linked to inference, it’s been established that one conversation with ChatGPT uses half a liter of water in terms of the data center cooling resources required (cf. the considerable heat generated by GPUs, above). Not to mention GPT-3’s training, which required 5.4 million liters of water (same source). That’s a bit more than one liter per training hour (training GPT-3 took 4.6 million GPU hours, according to… ChatGPT!)
Given these elements, it’s not surprising that AI energy demand is set to outpace supply.
If Google were to use AI for its around 9 billion daily searches - which it most likely will - it would need 29.2 terawatt hours (TWh) of power each year, according to researcher Alex de Vries. As such, as de Vries told Euronews last year, by 2027, AI could consume as much electricity as a medium-sized country like the Netherlands.
The IEA (International Energy Association) recently issued a similar warning: data centers’ energy consumption could more than double by 2026, to 1,000TWh, driven by AI and cryptocurrency.
One of AI’s most influential leaders naturally saw this coming: at Davos in January 2024, OpenAI CEO Sam Altman said AI will definitely need much more energy than initially thought. “There’s no way to get there without a[n energy] breakthrough [like nuclear fusion]”, Reuters reported him saying on a panel. This could well be why OpenAI’s most famous investor, Microsoft, just hired a new Director of Nuclear Development Acceleration: to “help power its own AI revolution”, according to TechRadar Pro.
Whilst we’re a long way off nuclear fusion - versus current fission methods - a trend of nuclear-powered data centers is definitely bubbling up.
According to AMD CEO Lisa Su, in around ten years’ time we may see zettaflop-class supercomputers, whose requirement for 500MW facilities will far outstrip todays’ 20-50MW facilities. Such needs can only be powered by local, dedicated sources like nuclear SMRs (small modular reactors).
This is why The Register reports that last year, Cumulus Data opened a 65MW nuclear data center, which it claims will ultimately reach a capacity of 950MW. In addition, SMR-powered facilities are currently being investigated by Green Energy Partners/IP3 (Virginia, USA) and Bahnhof (Sweden).
Given our current reliance on fossil fuels (e.g. with the US still dependent on them for 80% of its energy), could nuclear-powered emission-free data centers be a better option for the planet than current solutions? Time will tell, especially for future generations…
The first rule of any sustainability strategy, especially in tech, should be to ask “do I really need this?”
Indeed, generative AI is neither inevitable, nor adapted to all use cases. As we’ve already explained here, symbolic, or “good old-fashioned” AI, can do a lot more than what many of us expect, and with considerably less impact. French startup Golem.ai has notably established that one of their email-sorting symbolic AI models emits 1000 less CO2eq than GPT-3.
That said, if you do decide you absolutely must use generative AI, does it have to be on the scale of ChatGPT? Must it hoover up all of the world’s data, or can it just focus on a specialized dataset, like legal documents, for example?
Do you have to use a supercomputer for training, or would a smaller, single H100 GPU do the trick? Could you simultaneously prolong the life of old hardware and save money by using older generation GPUs?
For inference, could a less energy-hungry CPU, like Ampere’s, meet your needs (cf. above)?
Next, it can be inspiring to look into the many ways generative AI is being used today to actively further sustainability; potentially, to an extent that may far outweigh its impact.
Indeed, a McKinsey report once estimated AI-based technologies could help companies to reduce their emissions by up to 10%, and their energy costs by 10-20%.
One clear example in tech is Google’s AI subsidiary DeepMind, which declared as early as 2016 that its application of machine learning in GCP data centers has enabled said facilities to consume 40% less energy. How? By improving anticipation of key internal factors, like how different types of machinery interact with each other, and external ones like the weather, thanks to training data such as past temperatures, power, pump speeds and setpoints.
AI’s impact in data centers can also be reduced by using alternative cooling systems. This is the case of the DC5 data center, where Scaleway’s AI machines are housed, which consumes 30-40% less energy than standard facilities, because it uses no air conditioning. Instead, it relies on free cooling most of the year, and, in warmer summer months, adiabatic cooling, a process which cools outside air by passing it through a moist membrane. This, plus French energy’s low carbon intensity, makes DC5 one of the world’s least impactful AI installations.
Machine learning can also help in broader contexts, although many of today's LLM-based solutions are based more on predictive than generative AI. For example, using past data to predict future demand for electricity, thereby optimizing smart grids; anticipating road traffic, which can make travel, deliveries way more efficient, thereby reducing pollution (Google claims its Green Light initiative with Google Maps can reduce emissions at intersections by 10%); fine-tuning energy consumption in buildings via temperature prediction; and the forecasting of extreme weather events or incidents, like Pyronear, which uses AI-equipped towers to detect forest fires.
All of these examples and more - also covering societal impacts, public policy analysis, education and finance - are already happening thanks to generative AI. This white paper by leading academics and Google thought-leaders demonstrates how these diverse activities are accelerating sustainability as a whole.
Generative AI can also facilitate access to key information about sustainability. French national ecological agency (and Scaleway client) ADEME is currently experimenting with a text-based model trained on the agency's extensive documentation database, with the objective of extracting key data more quickly and understandably. If the experiment is successful, the model could be opened up to the general public. Spearhearded by Ekimetrics' "AI for Sustainability" team, the project is similar to "ClimateQ&A", a model trained on the IPCC reports, which is essentially a ChatGPT for sustainability (and not the only one, cf. this ChatGPT plugin, "IPCC Explainer").
Then there are flag-waving applications, which warn us of potential sustainability emergencies. Data for Good notably enabled ocean protection ONG Bloom to detect illegal fishing using AI, and used AI to power its Carbonbombs.org website, which flags the world’s most-polluting projects, such as coal mines, and is now influencing global policy. Finally, Climatetrace.org uses AI to highlight those countries that aren’t decarbonizing as quickly as they say they are.
Not forgetting the AI models themselves: the smaller they are, the less energy they consume, which makes them better for everyone, including the planet. As Mistral AI CEO and co-founder Arthur Mensch told ai-PULSE last November, “in the ‘Vanilla Attention’ version of Transformers, you need to keep the tokens in memory. “With Mistral AI’s ‘Sliding Window Attention’ model, there are four times less tokens in memory, reducing memory pressure and therefore saving money. Currently, too much memory is used by generative AI”. This is notably why the company’s latest model, Mistral-7B, can run locally on a (recent) smartphone.
Looking ahead, this efficiency-first approach will apply to AI solution offerings too. Scaleway aims to beta release by mid-2024 an inference service which will serve LLMs connected to clients’ private or sensitive data. Based on LLMs like Llama or those of Mistral AI, such a service is cost- and energy-efficient because:
If we consider that there are as many AI solutions as impacts, the jury is out at best. Most experts agree it’s too early in the generative AI revolution to measure its true impact on the planet.
But there is good news! Firstly, it is totally possible to assess impact before choosing the right AI model for your needs, namely:
Furthermore, the principles of green IT apply just as much to AI as they do to ‘traditional’ computing:
Last but not least, AI datasets, models and machines should only be as big or powerful as they need to be. Otherwise, tech’s eternal risk of falling into the rebound effect, or Jevon’s paradox - using a service more, rather than less, as it gets more efficient - could have dire consequences.
As Ekimetrics’ Head of AI for Sustainability Theo Alves Da Costa puts it, “if we use the bulldozer of AI to knock a nail into a wall, the nail will go in, but we also run the risk of knocking the whole wall down. In cases like this, it’s better to just use a hammer”.
Special thanks to Ekimetrics’ Theo Alves Da Costa for many of the sources in this article. And to Hugging Face’s Sasha Luccioni for the inspiration!
What is next for Green IT? Regulation, and 2050 carbon neutrality targets, will drive a shift from nice-to-have to must-have. And then there's AI...